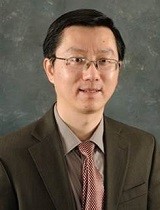
Huaiyu Dai
Professor, IEEE Fellow, Fellow of Asia-Pacific Artificial Intelligence Association
North Carolina State University
Title: Trilemma in Distributed Learning: Data Privacy, Communication Efficiency, and Accuracy
Abstract:
Distributed learning is envisioned to play an important role in next-generation AI-native wireless systems. By exploiting computing power and sensory data distributed across the network, this learning paradigm dramatically reduces communication burden and enhances data privacy, thus enabling network intelligence in a scalable manner. This talk focuses on the intriguing relationship of communication efficiency, data privacy and learning performance in the distributed training of deep neural networks. We first introduce our work on user training data reconstruction based on gradient information from the attacker's perspective, indicating that there is still a risk of data privacy leakage even if the principle of data minimization in distributed learning is followed. We examine and observe that different compressors vary in performance against the data reconstruction attacks. Then, to measure the privacy-preservation capability of gradient compression, we introduce our work on differential privacy of gradient compressors from the perspective of defensive mechanisms, revealing the inherent relationship between lossy compression and privacy protection.
Bio:
Huaiyu Dai received the B.E. and M.S. degrees in electrical engineering from Tsinghua University, Beijing, China, in 1996 and 1998, respectively, and the Ph.D. degree in electrical engineering from Princeton University, Princeton, NJ in 2002. He was with Bell Labs, Lucent Technologies, Holmdel, NJ, in summer 2000, and with AT&T Labs-Research, Middletown, NJ, in summer 2001. He is currently a Professor of Electrical and Computer Engineering with NC State University, Raleigh, holding the title of University Faculty Scholar. His research interests are in the general areas of communications, signal processing, networking, and computing, with over 280 peer-reviewed journal/conference papers published. His current research focuses on machine learning and artificial intelligence for communications and networking, multilayer and interdependent networks, dynamic spectrum access and sharing, as well as security and privacy issues in the above systems. He has served as an area editor for IEEE Transactions on Communications, a member of the Executive Editorial Committee for IEEE Transactions on Wireless Communications, and an editor for IEEE Transactions on Signal Processing. Currently he serves as an area editor for IEEE Transactions on Wireless Communications. He will serve as the Editor in Chief for IEEE Transactions of Signal and Information Processing over Networks in 2025. He was a co-recipient ofbest paper awards at 2010 IEEE International Conference on Mobile Ad-hoc and Sensor Systems (MASS 2010),2016 IEEE INFOCOM BIGSECURITY Workshop, and 2017 IEEE International Conference on Communications (ICC 2017). He received Qualcomm Faculty Award in 2019, and IEEE Communications Society William R. Bennett Prize in 2024. He is a Fellow of IEEE, and of Asia-Pacific Artificial Intelligence Association.
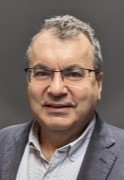
Title: Robust Multiplication-Free Kernels Related with L1-Norm and Haar Wavelet Based Network Layers
Abstract:
We describe a family of vector dot products that can be implemented using only sign calculations and addition/subtraction operations. The dot products are also energy-efficient because they do not perform energy consuming multiplication operations. Moreover, the vector products induce the ℓ1-norm. As a result, they are robust to outliers in data. We prove that some of the vector products satisfy Mercer's condition and yield symmetric, positive semi-definite generalized covariance matrices, thus enabling Kernel Principal Component Analysis (KPCA) and related energy-efficient signal and image processing applications. We can also use the new vector products in “convolution”-like operations in deep neural networks. In the second part of the talk, we will describe Haar Wavelet (or Hadamard transform) layers for neural networks to further reduce the energy consumption in edge applications. We will present practical Machine Learning applications. ML applications include (i) wildfire detection at the edge using cameras and (ii) sleep monitoring using infrared sensors.
Bio:
A.E. Cetin received his B.Sc. from METU, Ankara, Turkey, and Ph.D. in 1987 from the University of Pennsylvania, USA. He was an Assistant Prof. at the University of Toronto between 1987-1989 in Canada. He was a faculty member at Bilkent University from 1987-2017. He is currently a professor in the Department of Electrical and Computer Engineering at the University of Illinois at Chicago (UIC). He also has held visiting professor positions at Bellcore (1988), University of Minnesota (1996-1997), and UC San Diego (2016-2017). He has been carrying out research in the areas of theoretical and applied machine learning, signal, image, and video processing, biomedical signal processing, infrared and chemical sensor signal processing in Cyber-Physical Systems (CPS). His group introduced the concept of adaptive prediction and split vector quantization for Line Spectral Frequency representation. This concept was used in ITU speech coding standards including G.729, G.723.1 and GSM EFR. He became Fellow of IEEE for his contributions to signal and image recovery. He is the Editor-in-Chief of Signal, Image and Video Processing, Springer-Nature. He received a best paper award for his camera-based wildfire detection work in a conference organized by UNESCO and Cyprus Presidency of the European Union. He is one of the co-founders of the multinational smart wide angle OEM camera company Oncam-Grandeye (https://www.oncamgrandeye.com), UK. He served as the CEO/CTO of Grandeye, Turkey between 2003-2013. Oncam-Grandeye cameras won design and innovation awards in IFSEC, UK, and ISC WEST, Las Vegas, trade fairs.
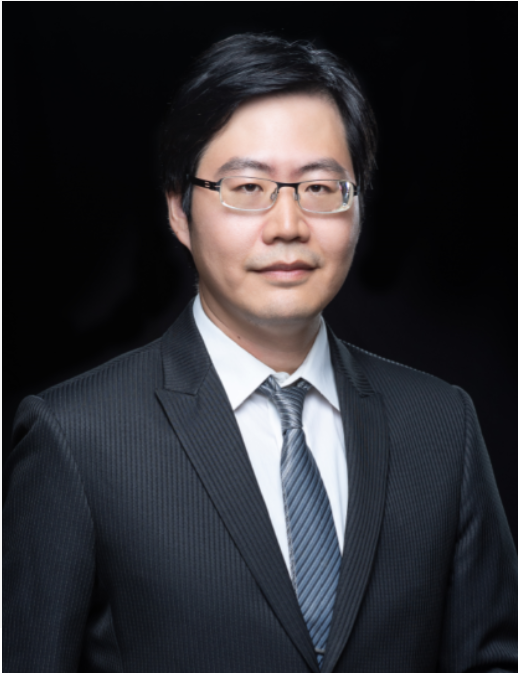
Tsung-hui Chang
Professor, IEEE Fellow
The Chinese University of Hong Kong, Shenzhen
Title: Aligning Model with Human Preference: A Ranking based Zeroth-order Optimization Method
Abstract:
In this study, we delve into an emerging optimization challenge involving a black-box objective function that can only be gauged via a ranking oracle—a situation frequently encountered in real-world scenarios, especially when the function is evaluated by human judges. A prominent instance of such a situation is Reinforcement Learning with Human Feedback (RLHF), an approach recently employed to enhance the performance of Large Language Models (LLMs) using human. We introduce ZO-RankSGD, an innovative zeroth-order optimization algorithm designed to tackle this optimization problem, accompanied by theoretical assurances. Our algorithm utilizes a novel rank-based random estimator to determine the descent direction and guarantees convergence to a stationary point. Last but not least, we demonstrate the effectiveness of ZO-RankSGD in a novel application: improving the quality of images generated by a diffusion generative model with human ranking feedback. Throughout experiments, we found that ZO-RankSGD can significantly enhance the detail of generated images with only a few rounds of human feedback. Overall, our work advances the field of zeroth-order optimization by addressing the problem of optimizing functions with only ranking feedback, and offers a new and effective approach for aligning Artificial Intelligence (AI) with human intentions.
Bio:
Tsung-Hui Chang is a Full Professor and Associate Dean (Education) at the School of Science and Engineering, The Chinese University of Hong Kong, Shenzhen, China, and Shenzhen Research Institute of Big Data. His research interests lie in optimization problems in data communications and machine learning. He is an Elected Member of IEEE SPS SPCOM TC and the Founding Chair of IEEE SPS ISAC TWG. He received the IEEE ComSoc Asian-Pacific Outstanding Young Researcher Award in 2015, and the IEEE SPS Best Paper Award twice in 2018 and 2021. He is currently a Senior Area Editor of IEEE TSP and an Associate Editor of IEEE OJSP. He is a Fellow of IEEE.
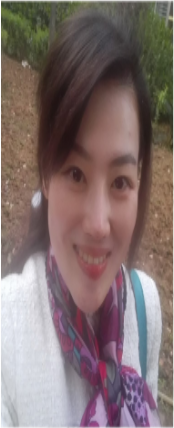
Title: On Partly Smoothness, Activity Identification and Faster Algorithms of L1 over L2 Minimization
Abstract:
The L1/L2 norm ratio arose as a sparseness measure and attracted a considerable amount of attention due to three merits: (i) sharper approximations of L0 compared to the L1; (ii) parameter-free and scale-invariant; (iii) more attractive than L1 under highly-coherent matrices. In this paper, we first establish the partly smooth property of L1 over L2 minimization relative to an active manifold M and also demonstrate its prox-regularity property. Second, we reveal that ADMMp (or ADMMp+) can identify the active manifold within a finite iterations. This discovery contributes to a deeper understanding of the optimization landscape associated with L1 over L2 minimization. Third, we propose a novel heuristic algorithm framework that combines ADMMp (or ADMMp+) with a globalized semismooth Newton method tailored for the active manifold M. This hybrid approach leverages the strengths of both methods to enhance convergence. Finally, through extensive numerical simulations, we showcase the superiority of our heuristic algorithm over existing state-of-the-art methods for sparse recovery.
Bio:
Tao Min's primary research focuses on the theory and application of optimization algorithms and optimization problems in machine learning. Her representative work has been published in flagship journals such as SIAM Journal on Optimization, SIAM Journal on Scientific Computing, SIAM Journal on Imaging Sciences, Mathematics of Computation, Journal of Scientific Computing, and the top computer science conference NIPS. In 2011, she received the Outstanding Young Paper Award from the Chinese Society for Computational Mathematics and was honored as an Outstanding Ph.D. Student in Jiangsu Province. In 2017, she won the Best Paper Award at the International Congress of Chinese Mathematicians. She has several highly cited articles, with one being listed as a hot paper in the field of Chinese science. She has led projects funded by the National Natural Science Foundation of China, including both Youth and General Programs, and a general project from Jiangsu Province. She has also participated in a national key research and development program. In 2018, she was selected as an Outstanding Backbone Teacher in the “Qinglan Project” for universities in Jiangsu Province. In 2022, she was named a Young and Middle-aged Academic Leader in the “Qinglan Project” for Jiangsu universities. She serves as a director of the Mathematical Programming Branch of the Operations Research Society of China, an invited reviewer for the American Mathematical Society, and a reviewer for top journals in optimization such as SIAM Journal on Scientific Computing and SIAM Journal on Optimization. Tao Min has conducted academic exchanges and research at the Chinese University of Hong Kong during September 2012-September 2013 with Prof. Raymond Chan, the University of Southern California during March 2016-May 2017 with Prof. JongShi Pang, and the University of Vienna during December 2022-December 2023 with Prof. Radu Ioan Bot.
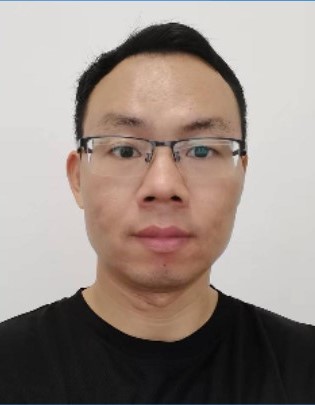
Title: DRM Revisited: A Complete Error Analysis
Abstract:
In this talk, we provide an answer to the question in deep Ritz Method (DRM) analysis under over-parameterization : Given a desired precision, how can we determine the number of training samples, the parameters of neural networks, the step size of gradient descent, and the number of iterations such that the output deep networks of the gradient.
Bio:
Yuliang Jiao is a professor at the School of Mathematics and Statistics, Wuhan University. His main research interests are in machine learning and scientific computing. His work has been published in journals and conferences such as SIAM J. Math. Anal., SIAM J. Control Optim., SIAM J. Numer. Anal., SIAM J. Sci. Comput., SIAM J. Math. Data. Sci., Appl. Comput. Harmon. Anal., J. Mach. Learn. Res., IEEE Trans. Inf. Theory, Ann. Stat., J. Amer. Statist. Assoc., Statist. Sci., Inverse Probl., IEEE Trans. Signal Process., ICML, and NeurIPS.
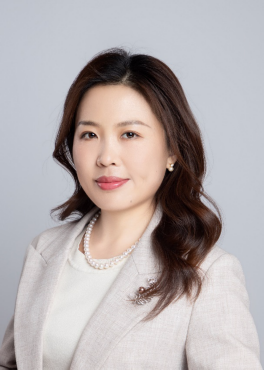
Title: Large-scale 3D Neuron Reconstruction and Morphology Analysis from Electron Microscopy Images
Abstract:
With the advent of advanced serial section electron microscopy (EM) imaging techniques, several EM data of mm3-scale volumes have been published for the connectomics community, with the data sizes ranging from terabytes to petabytes. The current neuron reconstruction pipeline for EM data usually includes automatic image segmentation followed by extensive human expert proofreading. Our work aims to reduce human workload by predicting connectivity between over-segmented neuron pieces, taking both microscopy image and 3D morphology features into account, similar to human proofreading workflow. In this talk, I will introduce our recently proposed deep learning approaches for morphological analysis for neuron classification and retrieval, connectivity prediction of neuron segments, and long-range neuron 3D tracing.
Bio:
Xuejin Chen is a professor with the National Engineering Laboratory for Brain-inspired Intelligence Technology and Application, University of Science and Technology of China. She received her B.S. degree and Ph.D. degree in electronic circuits and systems from University of Science and Technology of China, in 2003 and 2008 respectively. From 2008 to 2010, she conducted research as a postdoctoral scholar in the Department of Computer Science at Yale University. She was a visiting scholar at Microsoft Research Asia and Stanford University in 2015 and 2017 respectively. Her research interests include 3D modeling, geometrical analysis, and biological image analysis. She has authored or co-authored over 80 articles in top journals and conferences (including ACM TOG, IEEE TMM, ACM SIGGRAPH, Multimedia, ICCV, NeurIPS). She was one recipient of the Honorable Mention Awards of CVM Journal in 2019, Best papers of ICME Workshop 2024, GDC 2022, and the first prize of XPRESS challenge in ISBI 2023.
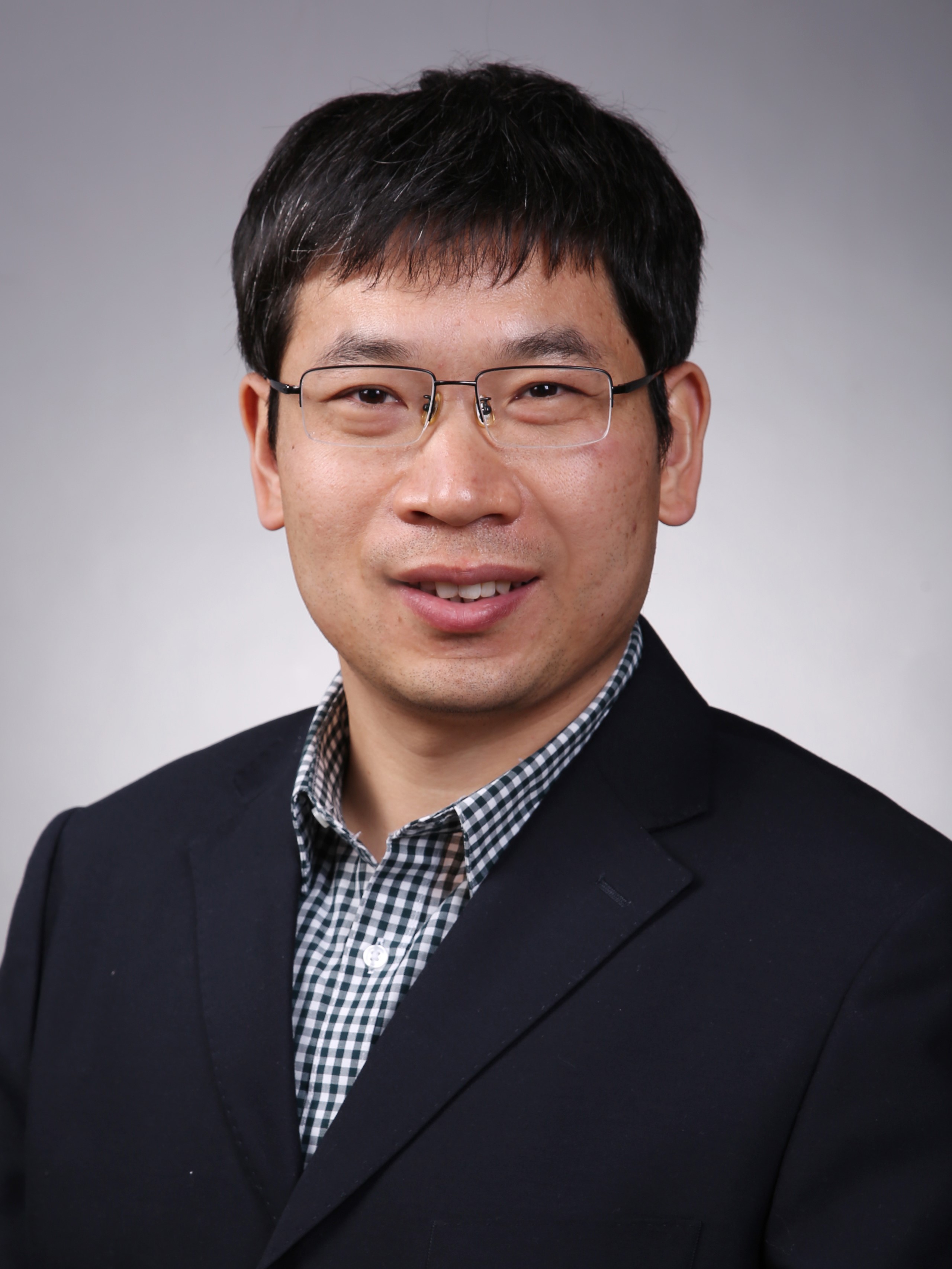
Ya-Feng Liu
Associate Professor
Academy of Mathematics and Systems Science, Chinese Academy of Sciences
Title: Covariance-Based Activity Detection in Cooperative Multi-Cell Massive MIMO: Scaling Law and Efficient Algorithms
Abstract:
In this talk, we focus on the activity detection problem in a multi-cell massive multiple-input multiple-output (MIMO) system, where active devices transmit their signature sequences to multiple base stations (BSs), and the BSs cooperatively detect the active devices based on the received signals. In this talk, we shall present some recent results of the covariance-based approach for activity detection in cooperative multi-cell massive MIMO systems. In particular, we shall present some scaling law results and efficient coordinate descent (CD) algorithms.
Bio:
Ya-Feng Liu (M'12--SM'18) received the B.Sc. degree in applied mathematics from Xidian University, Xi'an, China, in 2007, and the Ph.D. degree in computational mathematics from the Chinese Academy of Sciences (CAS), Beijing, China, in 2012. During his Ph.D. study, he was supported by the Academy of Mathematics and Systems Science (AMSS), CAS, to visit Professor Zhi-Quan (Tom) Luo at the University of Minnesota (Twins Cities) from 2011 to 2012. After his graduation, he joined the Institute of Computational Mathematics and Scientific/Engineering Computing, AMSS, CAS, Beijing, China, in 2012, where he became an Associate Professor in 2018. His main research interests are nonlinear optimization and its applications to signal processing, wireless communications, and machine learning. Dr. Liu currently serves as an Associate Editor for the IEEE Transactions on Signal Processing, and the Journal of Global Optimization, and as a Lead Guest Editor for the IEEE Journal on Selected Areas in Communications special issue on “Advanced Optimization Theory and Algorithms for Next Generation Wireless Communication Networks”. He served as an Editor for the IEEE Transactions on Wireless Communications (2019--2022) and an Associate Editor for the IEEE Signal Processing Letters (2019--2023). He is an elected member of the Signal Processing for Communications and Networking Technical Committee (SPCOM-TC) of the IEEE Signal Processing Society (2020--2022 and 2023--2025). He received the Best Paper Award from the IEEE International Conference on Communications (ICC) in 2011, the Chen Jingrun Star Award from the AMSS in 2018, the Science and Technology Award for Young Scholars from the Operations Research Society of China in 2018, the 15th IEEE ComSoc Asia-Pacific Outstanding Young Researcher Award in 2020, and the Science and Technology Award for Young Scholars from the China Society for Industrial and Applied Mathematics in 2022. Students supervised and co-supervised by him won the Best Student Paper Award from the International Symposium on Modeling and Optimization in Mobile, Ad Hoc and Wireless Networks (WiOpt) in 2015 and the Best Student Paper Award of IEEE International Conference on Acoustics, Speech and Signal Processing (ICASSP) in 2022.
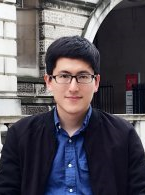
Title: Robot Embodiment through Visuo-Tactile Intelligence
Abstract:
This talk explores the important role of visuo-tactile intelligence in shaping robot embodiment, enabling their interactions with the physical world. By integrating visual and tactile sensory modalities, robots attain a deeper understanding of their surroundings, facilitating perception for agile grasping and manipulation. Drawing upon recent advancements in tactile sensor development including GelTip, TouchRoller and GelFinger, multimodal integration, and simulation techniques, I will delve into the foundations of visuo-tactile learning and its implications for robotics. Through case studies and experimental insights, I will share how this paradigm shift in robotic perception enhances adaptability, dexterity, and autonomy.
Bio:
Dr Shan Luo is a Reader (Associate Professor) in Robotics and AI at King’s College London. He received his PhD in Robotics from King's College London in 2016. Shan visited the MIT Computer and Artificial Intelligence Laboratory (CSAIL) in 2016. After completing his PhD, he worked as a Postdoctoral Research Fellow at the University of Leeds and Harvard University. Shan joined the University of Liverpool as a Lecturer in 2018 and was the Director of the smARTLab at the Department of Computer Science between 2018-2021. His research outputs have been published in high-impact robotics journals and international conferences, including Autonomous Robots, IEEE/ASME Transactions on Mechatronics, ICRA, IROS, RSS, ECCV, ICML and AAMAS. He has been a Guest Editor for IEEE Transactions on Robotics and Robotics and Automation Magazine, and an Associate Editor for ICRA and IROS. His research has received funding from prestigious funding bodies and industrial support including EPSRC, AHRC, Innovate UK, Royal Society and Unilever, with him as a PI for over £1.3 million. Shan's accolades also include the EPSRC New Investigator Award, BCS Academy New Appointments Grant Scheme Award, UK-RAS Early Career Award, Faculty Learning & Teaching and Student Experience Award.
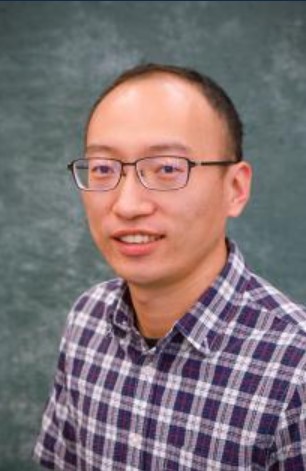
Title: Generative Image Reconstruction Using Diffusion Models: A Paradigm Shift from Vanilla Sparsity to Generative Modeling
Abstract:
Image reconstruction tasks such as super-resolution, denoising, deblurring, colorization, and compressed sensing are critical in computer vision applications. These tasks often involve solving ill-posed inverse problems due to incomplete or noisy observations, requiring the incorporation of prior knowledge about the image structure. Traditionally, this prior knowledge has been incorporated through sparsity-based methods, which assume that the target image can be represented by a sparse set of basis functions. While effective, sparsity-based approaches often struggle with complex image structures and might fail to capture the full richness of natural signals. Diffusion models (DM), also known as score-based generative models (SGM), offer a new paradigm by leveraging their powerful distribution modeling capabilities. Unlike sparsity-based methods, diffusion models can learn and represent the intricate structural features of natural images. As a result, by using diffusion models as an implicit prior and applying the Bayesian principle for posterior inference, we can achieve more effective image reconstruction. This talk is structured into three parts: The first part provides a concise overview of image reconstruction and sparsity-based solutions, followed by a tutorial introduction to diffusion models. The second part focuses on linear image reconstruction problems, presenting a diffusion model-based posterior sampling algorithm (DMPS). The final part addresses nonlinear image reconstruction problems, specifically quantized compressed sensing (QCS), introducing a QCS algorithm based on SGM (QCS-SGM) and its enhanced version QCS-SGM+, suitable for both row-orthogonal and general measurement matrices.
Bio:
Dr. Xiangming Meng is an assistant professor at The Zhejiang University-University of Illinois Urbana-Champaign Institute (ZJUI), Zhejiang University. He received his Bachelor's degree in Communication Engineering from Xidian University in 2011 and his Ph.D. in Information and Communication Engineering from Tsinghua University in 2016. From 2016 to 2019, he worked as a Senior Research Engineer at Huawei Technologies Co., Ltd. in Shanghai. He then pursued postdoctoral research from 2019 to 2020 at the RIKEN Center for Advanced Intelligence Project (AIP) in Japan, and from 2020 to 2022 in the Department of Physics at the University of Tokyo. From April 2022 to March 2023, he served as a Project Assistant Professor at the University of Tokyo. In March 2023, he joined the ZJU-UIUC Institute at Zhejiang University. Dr. Meng's research interests lie at the intersection of machine learning, signal processing, and statistical physics, with a focus on probabilistic machine learning, approximate Bayesian inference, and learning algorithms. Recently, he has shown particular interest in diffusion models and their applications in various inverse problems such as compressed sensing and image reconstruction. His work has been published as first author in top international journals and conferences, including NeurIPS, ICML, ICLR, AAAI, AISTATS, and IEEE JSAC. Dr. Meng has been invited to serve as an Area Chair for NeurIPS and as a reviewer for several prestigious journals and conferences, including Statistics and Computing, IEEE TCOM, NeurIPS, ICML, ICLR, CVPR, AAAI, and AISTATS, among others. He was also recognized as an Outstanding Reviewer at ICLR 2022.
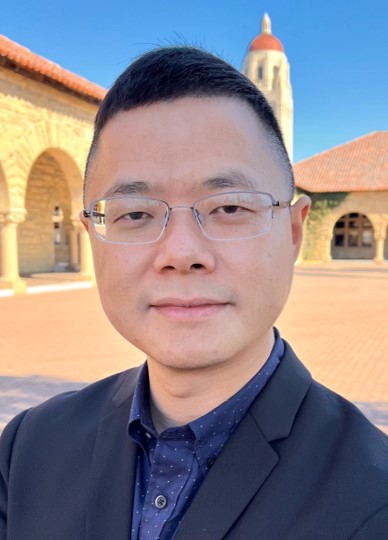
Title: InfoBodied AI: Learning Mutual Information for Embodied AI
Abstract:
Embodied AI strives to create agents capable of learning and tackling complex tasks involving physical interactions, with potential applications in many areas, such as housekeeping, caregiving, and logistics. Such agents must be able to perceive their environment, construct scene representations, and carry out reasoning and actions to accomplish task-specific goals. However, existing learning approaches rely on human annotations or unrealistic simulations, leading to generalization problems in the real world. Thus, it is crucial to equip embodied agents with the ability to autonomously learn from real-world data, minimizing reliance on human supervision and enabling adaptability to new tasks. We propose that the key to autonomous learning of embodied agents is the mutual correlations in the unlabeled data. In this presentation, we will talk about how we can efficiently compute mutual information of data by developing novel neural estimators. We will also show how these freely available mutual correlations can help reduce human annotation effort in learning label-efficient perception, scene representation, and grounded concepts for generalizable interaction policies. Finally, we show a potential framework to build embodied agents that can learn in unseen environments and automatically acquire novel interaction skills by leveraging mutual information in unlabeled observational data.
Bio:
Yanchao Yang is an Assistant Professor at HKU with the Department of Electrical and Electronic Engineering and the HKU Musketeers Foundation Institute of Data Science (HKU-IDS). He does research in Embodied AI and is interested in self-supervised and semi-supervised techniques that allow autonomous agents to learn at low-annotation regimes. His long-term goal is to design learning algorithms that enable autonomous agents to continuously acquire object-centric representations through active perception with multimodal signals for compositional scene understanding and reasoning. His recent effort is to leverage information-theoretic tools to promote label-efficient perception, scene representation, and policy learning for embodied intelligence.